It’s an exciting time in the world of AI Foundation tools and utilities. Every day, new advancements in core models, new performance benchmarks, new purpose-built tools and more comprehensive APIs emerge. It can be a challenge to stay on top of the pace of change, but the AI Foundation and all the providers in that space are critical building blocks to true AI Solutions.
In addition to the AI Foundation, true AI Solutions, meaning actual functional line-of-business value, are also dependent on AI Enablement layers. AI Enablement includes traditional software-driven workflow, model orchestration and system integration. Integration often spans enterprise systems and data sources and third party or external data sources or utilities. Fully assembled, the combination of utilities, models, software functionality, integrations and human-AI interfaces deliver viable and ROI-driving AI Solutions.
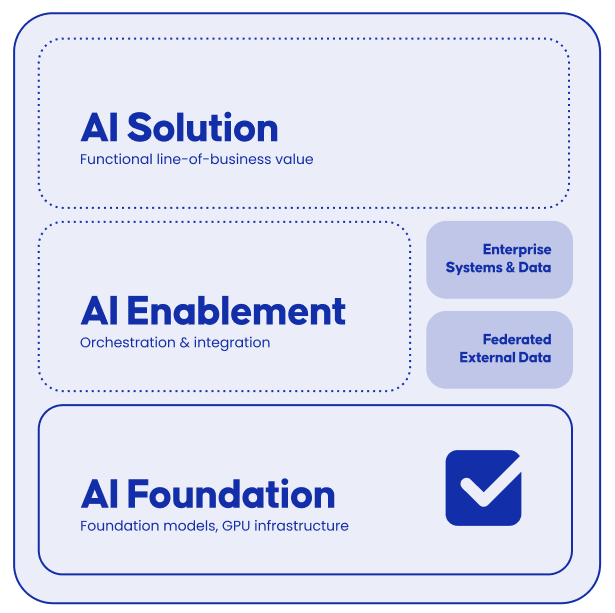
Unlike a traditional building foundation though, modern AI Solutions need to be adaptable to a changing foundation. They can’t collapse in response to substantial foundation change. For instance, Meta’s recent announcement of Large Concept Models (LCMs) as an alternative to Large Language Models (LLMs) presents. As Amir Zeinali notes in this LinkedIn post,
“Meta’s new Large Concept Models (LCMs) flip the script by operating at the sentence level in a language-agnostic, high-dimensional embedding space. Instead of token-based predictions, LCMs use “concepts” (think semantic ideas, not just words)”
This shift might fundamentally change the level and structure of AI prompting, the type and depth of context (and size of context window) you need to provide or account for, the complexity of a traditional query+response and the nature of how you have to handle an AI response within a given AI Solution. If your system is ‘hard-wired’ into an existing LLM and lacks the appropriate AI Enablement extraction layer, that treats the foundation as a toolkit to be implemented and optimized, you may find yourself stuck.
The better approach is to build your AI Solution on purpose-built platform like Contextual, where you can Own Your AITM by creating the workflow, logic, data management, data enrichment and AI model processing required at an abstraction above the underlying AI Foundation. That way modifying prompts, adjusting the context window shared and processing responses supports rapid adjustment and modification of the AI Foundation layer changes.
Further, if you’re thinking about using AI in your enterprise, you have to be prepared for coming pricing and costing wars. Companies like OpenAI cannot continue to burn through piles of money indefinitely to subsidize every request for a new cat haiku or funny AI-generated picture of a platypus. Just recently Futurism published an article indicating OpenAI’s latest model can cost up to $1,000 per query. Sweet mother of pearl! The reasoning of models like o3 can be truly breathtaking, but your average AI Solution cannot justify that type of cost.
If you’re using AI to simply augment or enrich existing processes, tasks and functions, the ROI you can drive is tightly dependent on the costs of the system. You may be actively seeking lower and lower per-AI transaction or ultimately unlimited AI transaction systems to enable your solution. This is one reason Contextual delivers such a high level of transparency, where cost for the system is tied directly to the processing required to run your AI Solution. There are no hidden per seat or per user costs, but rather you can directly correlate your AI Solution’s unit of work (for instance quotes produced) to the system cost itself.
Ultimately, the pace of change and the risk of costs in the AI Foundation absolutely necessitate that thoughtful businesses future-proof their organizations and their AI Solutions by owning their own AI systems. By building on a transparent stack like Contextual with simplicity of swapping between AI Foundation utilities, a business can ensure their AI approach drives the best ROI with minimal risk.